Scientists and researchers around the world are reaching an entirely new level of discovery with Artificial Intelligence (AI), using data to solve problems and enabling decisions in innovative new ways. AI remains a dominant conversation at scientific symposia across the globe and once again received top billing at RSNA and ISMRM annual meetings. One need not travel far to see how AI is creating new frontiers – it is also a big part of the research being conducted at the University of Wisconsin Department of Radiology.
The term AI dates back to the 1950s, but its present state is possible through the development of supercomputers. Advances in computer hardware over the past decade have paved the way for very complex algorithms to run quickly and easily, producing data faster than we could ever have imagined before. The Department has made the commitment to create a robust technical infrastructure to support innovation and encourage the expansion of AI in Radiology here at UW. Our Director of Informatics, John Garrett, PhD, is currently focusing on developing the infrastructure to support faculty and provide access to the type of equipment needed to process the large amounts of data necessary for Deep Learning (DL), a subset of AI.
The department invested in a GPU supercomputer, a networked group of computers whose processing power far surpasses the capabilities of even the most powerful CPU. “These are the same computers used in gaming applications,” Garrett said. “The power of the GPU processing is equivalent to a whole server farm – this is the type of computer we want to get people used to using to be able to do things they simply can’t on other computers,” he said.
Dr. Garrett noted that there are challenges to incorporating AI into a traditional hospital network, and to be able to integrate it into the clinical workflow. That is where many of the department faculty are closing the gap.
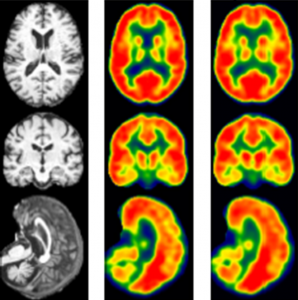
Vivek Prabhakaran, MD, PhD, is Department of Radiology faculty member who is using AI to make new discoveries. He has led the efforts to train an AI model using MRI input to create synthetic FDG PET images. Traditional FDG PET scans are currently the standard for understanding brain metabolism and diagnosing Alzheimer’s disease. They involve the injection of a radioactive tracer, fluorodeoxyglucose, or FDG, into the patient before the PET scan, to show the differences between the healthy and diseased tissue in the brain. Dr. Prabhakaran’s synthetic version using MRI involves no radiation and had a 97 percent correlation rate with the test that used radiation. “Not only is this new method non-invasive, without the injection of radioactive tracers, but it can be done at a much lower cost,” Dr. Prabhakaran said. The AI algorithm used by Prabhakaran has great potential to be expanded to other uses, for example to help diagnose cancer, epilepsy and myocardial viability.
Alan McMillan, PhD, director of the Molecular Imaging / Magnetic Resonance Technology Lab (MIMRTL) is one of “superusers” of the NVIDIA® DGX™ supercomputer system, seeking ways to advance MRI, PET/MR and PET/CT imaging techniques. “There is no aspect of our research that doesn’t incorporate AI,” McMillan said. In fact, he sees the rapid growth of AI as a boon to all future research, allowing machines to focus on the algorithms and enabling people to focus on the more physical aspects of human health. Some of McMillan’s recent efforts have centered on finding a way to create CT-like imaging from MRI images. Using Deep Learning, Dr. McMillan’s team has been able to reconstruct robust CT images directly from clinical MR images. When these images are used to perform attenuation correction, they enable more quantitatively accurate PET images for simultaneous PET/MR and reduce error from 10-25% (in conventional techniques) to less than 5%.
Another researcher and clinician who is tapping into AI to discover new information is Perry Pickhardt, MD. Dr. Pickhardt recently found a new way to obtain additional “opportunistic” diagnostic information about the structures seen in the abdomen during abdominal Computed Tomography (CT) imagery performed during CT colonography. “CT sees many things,” Dr. Pickhardt said. “We wanted to take the extra biomarkers in the CT scans and try to do something more with them. A CT scan also has information about patients’ bone, muscle, fat, calcium, and more,” Pickhardt continued. “With this, we can get a read on many other important health connotations, for example, we can quantify the aortic calcium levels of a patient and make a prediction about his or her likelihood of a cardiac event.” Dr. Pickhardt’s paper, “Fully-Automated Analysis of Abdominal CT Scans for Opportunistic Prediction of Cardiometabolic Events: Initial Results in a Large Asymptomatic Adult Cohort,” received the Roscoe E. Miller Best Paper Award at the recent Society of Abdominal Radiology (SAR) conference.
Vivek Prabhakaran, MD, PhD, is another Department of Radiology faculty member who is using AI to make new discoveries. He has led the efforts to train an AI model using MRI input to create synthetic FDG PET images. Traditional FDG PET scans are currently the standard for understanding brain metabolism and diagnosing Alzheimer’s disease. They involve the injection of a radioactive tracer, fluorodeoxyglucose, or FDG, into the patient before the PET scan, to show the differences between the healthy and diseased tissue in the brain. Dr. Prabhakaran’s synthetic version using MRI involves no radiation and had a 97 percent correlation rate with the test that used radiation. “Not only is this new method non-invasive, without the injection of radioactive tracers, but it can be done at a much lower cost,” Dr. Prabhakaran said. The AI algorithm used by Prabhakaran has great potential to be expanded to other uses, for example to help diagnose cancer, epilepsy and myocardial viability.
Innovations made possible by AI are occurring in practically every area of the imaging world. Department of Radiology researchers Richard Kijowski, MD, and Fang Liu, PhD, have developed a fully automated deep-learning system that uses two deep convolutional neural networks to detect anterior cruciate ligament ears (ACL) tears on knee MRI exams. The result of their research showed that a fully automated deep learning network could determine the presence or absence of ACL tears with similar diagnostic performance as experienced musculoskeletal radiologists. Similar deep learning algorithm have been developed by Drs. Kijowski and Liu to detect other musculoskeletal pathology, including cartilage lesions on MRI and hip fractures on pelvic radiographs. The use of deep learning methods to detect musculoskeletal pathology could provide immediate preliminary interpretations of imaging studies, maximize diagnostic performance, and reduce errors due to distraction and fatigue. “However, future work is needed for further technical development and validation before this could be implemented into clinical practice,” said Dr. Kijowski. “I look forward to the future of this research.”
The evolution of AI is currently thriving across UW campus, and is especially evident in the Department of Radiology. But many are wondering, what should we expect from the future of AI? “My hope for AI is that it will create a better use for people’s intelligence,” Dr. McMillan said. “We can decide what problems are most important, and use AI to solve problems in faster and better ways. For many scientists, AI need not be the focus of their research, rather it can be leveraged as a tool that enables us to solve problems that couldn’t easily be solved in any other way,” he said. “The hope for the future is that we will keep finding more hard problems for AI to solve more quickly and efficiently. It is truly humbling and exciting work.”