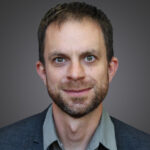
Kevin Johnson, PhD received a five-year, $3.5 million grant for “Pediatric Cardiopulmonary MRI using RF Navigators and High Dimensional Deep Learning” from the National Institutes of Health/National Heart, Lung, and Blood Institute. This project aims to develop MRI technology to make it easier to image the cardiac and pulmonary systems in pediatric patients without sedation or radiation.
Imaging the cardiac and pulmonary systems in pediatric patients presents challenges. MRI scans are highly sensitive to motion, and young patients often struggle to remain still for the duration of the scan. As a result, captured scans may be unclear and contain motion artifacts like blurring or ghosting. To obtain accurate imaging in a clinical setting, pediatric patients are often sedated before the scans; however, this is not always a viable option.
Dr. Johnson is collaborating with colleagues at UW–Madison; Stanford University; University of California, Berkeley; and University of California, San Francisco to develop a dual-pronged method to solve the challenge of capturing pediatric MRI scans. The first involves fast imaging techniques to reduce the overall time it takes to capture scans.
The second approach measures motion during the scans. The team developed a unique method that transmits a Wi-Fi (5 GHz) signal through the body, tracking interference caused by the patient. The signal changes pattern with bulk motion, breathing, and beating of the heart; effectively measuring the amount of motion during a scan. This information is then harnessed in a high dimensional deep learning approach, which applies 2D and 3D artificial intelligence (AI) technologies to high resolution 4D applications. Ultimately, this allows scans that have been disturbed by motion to be reconstructed.
Dr. Johnson noted “We hope that this grant will push MRI closer to a more push-button method, closer to that of CT. This particular project will target the imaging of congenital heart disease, providing extremely valuable information for treatment and monitoring.”
Current cardiopulmonary evaluations typically require multiple exams to evaluate the cardiovascular and pulmonary systems; which can involve complex logistics and delay patient care. The combination of increased MRI speed and ability to reconstruct images via deep learning would expedite the treatment and reduce stress for the patients.
While this project is using imaging techniques for pediatric patients with congenital heart disease, the increased MRI speed and image reconstruction methods could potentially be expanded to capture accurate imaging for other motion-related imaging issues.