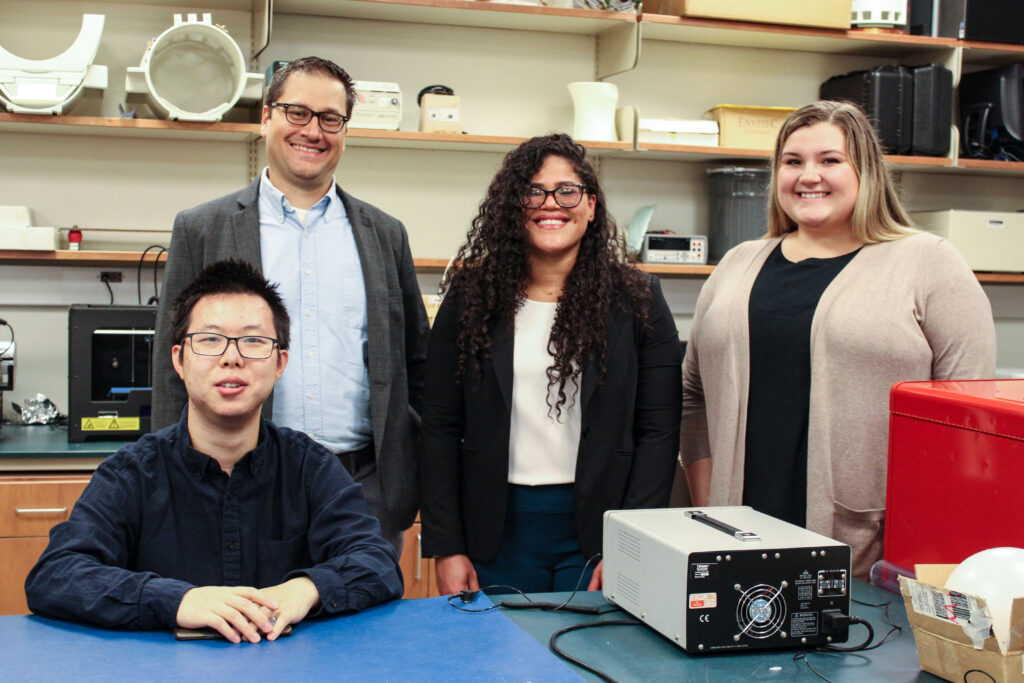
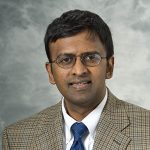

Mentoring is a key focus of the Department of Radiology, with our faculty mentoring students and trainees of all levels. This includes research mentorship, and we are proud to have the research mentees of three faculty – Alan McMillan, PhD, Vivek Prabhakaran, MD, PhD, and Reinier Hernandez, PhD – present at the UW-Madison Undergraduate Research Symposium. As well, Dr. Alan McMillan was awarded the 2021-22 URS Exceptional Mentor award from the Undergraduate Research Scholars (URS) program.
The 24th Annual Undergraduate Research Symposium was held virtually on April 28 and 29, 2022. The purpose of the Symposium is to “showcase undergraduate creativity, achievement, research, service-learning and community-based research from all areas of study at UW–Madison.” Learn more about the projects presented at the Symposium below:
Analysis of Probabilistic Classifiers for Disease Detection in Medical Imaging
Camilla Appiani, Alan McMillan (Mentor)
Project Description: The medical field is constantly growing with regards to new applications of artificial intelligence and machine learning. Current implementations include using neural networks with multiple layers to classify medical scans, to suggest diagnoses from medical images. This project analyzes probabilistic classifiers that associate a confidence rating to diagnostic classifications, where our goal is to understand how reliable and consistent probabilistic classifiers are. Using large datasets of chest x-ray images, we aim to test deep generative and Monte Carlo dropout classifiers and compare their diagnostic performance. If successful in demonstrating the consistency of probabilistic classifiers, it will promote further work to build and implement probabilistic classifiers in automated disease detection from medical images.
MRI Features of Immunotherapy Use in Lung Cancer Brain Metastasis Patients
Chengnan Li, Alan McMillan (Mentor)
Project Description: Immunotherapy treatments like immune checkpoint inhibitors (ICIs) are now routinely used for treatment of metastatic non-small cell lung cancer (NSCLC). Brain MRI changes in response to ICI and stereotactic radiosurgery (SRS) can sometimes be challenging to interpret. This project uses radiomic analysis to objectively characterize the differences between NSCLC brain metastases treated with and without immunotherapy after SRS. Post-SRS radiomic features were analyzed with a machine learning model using extreme gradient boosting algorithms to predict the presence of immunotherapy treatment. Preliminary results yielded a receiver operator curve AUC of 0.84 ± 0.12, but will be expanded on with more patients and MR imaging modalities. Radiomics and machine learning analysis can provide insight into assessing effectiveness of immunotherapy treatments and predicting patient outcomes.
Artificial Intelligence and Deep Learning in Medical Imaging
Paridhi Gupta, Alan McMillan (Mentor)
Project Description: Many diseases are time-sensitive, i.e., they can become much worse and even fatal if they do not get detected and treated in time. Detecting them using medical images requires careful observations, which takes a lot of focused time. This research aims to speed up the analysis process of whole body images, making it more reliable, efficient and accurate. It aims to use deep learning techniques to create an artificial intelligence to analyze and detect abnormalities and diseases from segmentations of medical images. It builds on the previously created technique of training Convolutional Neural Networks (CNN) to identify organs and abnormalities in from whole body images. The program trains, validates, tests, and then provides its accuracy rate for each organ.
Efficacy of Brain-Computer Interfaces and Functional Electrical Stimulation Intervention on Outcome Measures of Hand Motor Function in Stroke Survivors
Thomas Hosseini, Vivek Prabhakaran (Mentor)
Project Description: Stroke motor impairment can be treated by non-invasive electroencephalographic (EEG)-based brain-computer interfaces (BCI). We sought to determine whether BCI+functional electrical stimulation (FES) improves motor function.21 stroke survivors (f = 16, age = 61.69±13.07, chronicity at baseline = 33.15±41.84 months) with motor impairment completed 4–32 hours of BCI-FES intervention. Group averages of hand grip strength (HGS) and Nine Hole Pegboard Test (NHPT) were compared at baseline and completion. Participants showed adaptive change from pre-intervention to post-intervention (HGS: PRE 16.07±19.67 lbs, POST 19.31±21.47 lbs, p = 0.01) (NHPT: PRE 184.65±128.91 seconds, POST 183.66±129.51 seconds, p = 0.92). Results indicate that BCI-FES intervention can improve post-stroke hand function.
Testing Broad Spectrum Cancer Therapeutics in Murine Models
Amanda Carston, Reinier Hernandez (Mentor)
Project Description: The goal of this research is to discover how radiolabeled NM600, a tumor-targeting agent, may serve well as both therapeutic and diagnostic tools to provide targeted and personalized cancer treatments. Current radiotherapy is typically successful in eradicating cancer at the cost of exposing healthy tissue to radiation, which may cause adverse events. Murine cancer models are used to test the efficacy of these radioligands through in-vivo and in-vitro techniques. If found viable, this powerful technique does not only have anti-tumor effects, but it may also prevent excessive radiation exposure to healthy tissues by only attacking the tumor cells, which would reduce the severity of unwanted side effects cancer patients tend to suffer from.