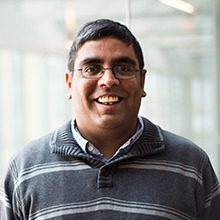
Speaker: Vikas Singh (Biostats)
Seminar Title: Visual Relations, Relative Attributes and Graph Neural Networks
Date: August 3
Time: 4pm-5pm
Location: SMPH/HSLC Room 1325
Abstract: Exploiting relations between entities within an image or between samples in a set of training examples can be very useful in a number of image interpretation and analysis tasks in computer vision. This can be accomplished using shallow models, deep architectures and/or hybrid formulations that mix and match concepts from both. In this talk, I will describe some of our recent results focused on leveraging such “relational” information for improving the performance of computer vision algorithms for visual relationship learning, semi-supervised learning and relative attribute learning. In this talk, we will first cover the simpler settings where the ideas offer impressive results on standard computer vision problems which provide large training datasets. Then, we will discuss preliminary results on the (more challenging) use cases in medical image analysis including design of clinical trials with moderately sized datasets. If time permits, we will see an overview of some ongoing projects based on extensions/variants of these concepts.
Speaker Bio: Vikas Singh is a Professor in the Department of Biostatistics & Med. Info and Computer Sciences (affiliate). Singh and his students are engaged in algorithmic and applications-motivated research in computer vision, image analysis and machine learning with a special focus on problems in biomedical image analysis. He teaches classes in AI, image analysis and computer vision.